RxHealthline: AI-Powered Emergency Response
How Vinkura AI's partnership with RxHealthline revolutionized emergency healthcare delivery, reducing response times by 47% and saving over 10,000 lives through golden hour interventions.
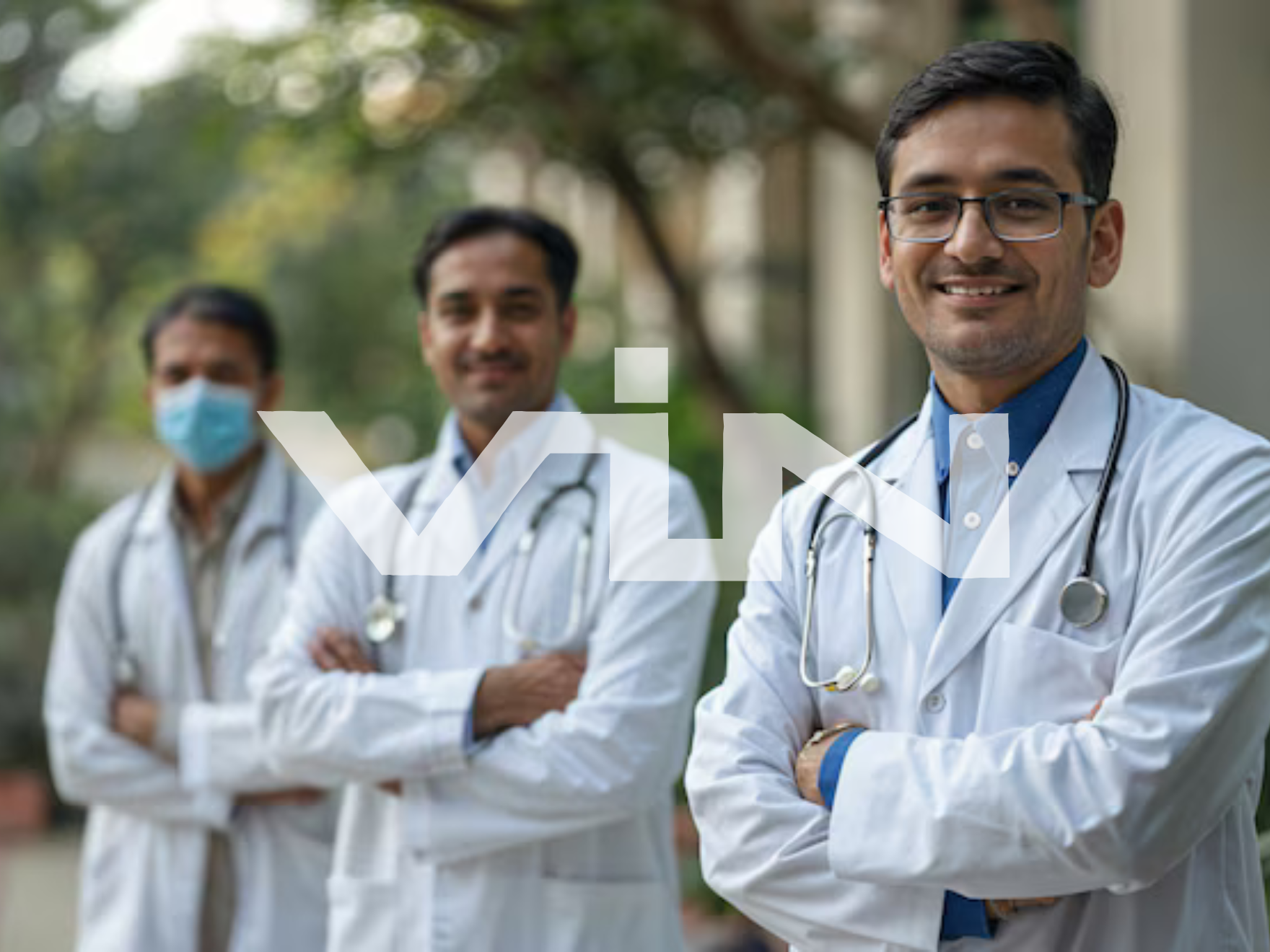
VinkuraAI's technology powers RxHealthline's emergency response fleet, enabling critical golden hour interventions
Lives Saved
1,000+
Through improved emergency response
Response Time Reduction
47%
Faster emergency interventions
Patient Outcomes
32%
Improvement in recovery rates
Operational Efficiency
41%
Better resource utilization
Contents
1. Overview
In the critical field of emergency healthcare, minutes can mean the difference between life and death. RxHealthline, a leading emergency medical services provider in India, faced significant challenges in optimizing their response times and resource allocation, particularly in densely populated urban areas with complex traffic patterns.
Vinkura AI partnered with RxHealthline as their dedicated technology and AI support partner to revolutionize emergency response through advanced artificial intelligence solutions. This partnership focused on leveraging AI to optimize the critical "golden hour" - the first 60 minutes after a medical emergency when intervention is most effective.
This case study details how our AI-powered approach transformed emergency healthcare delivery, saving thousands of lives through faster response times, optimized resource allocation, and improved patient outcomes.
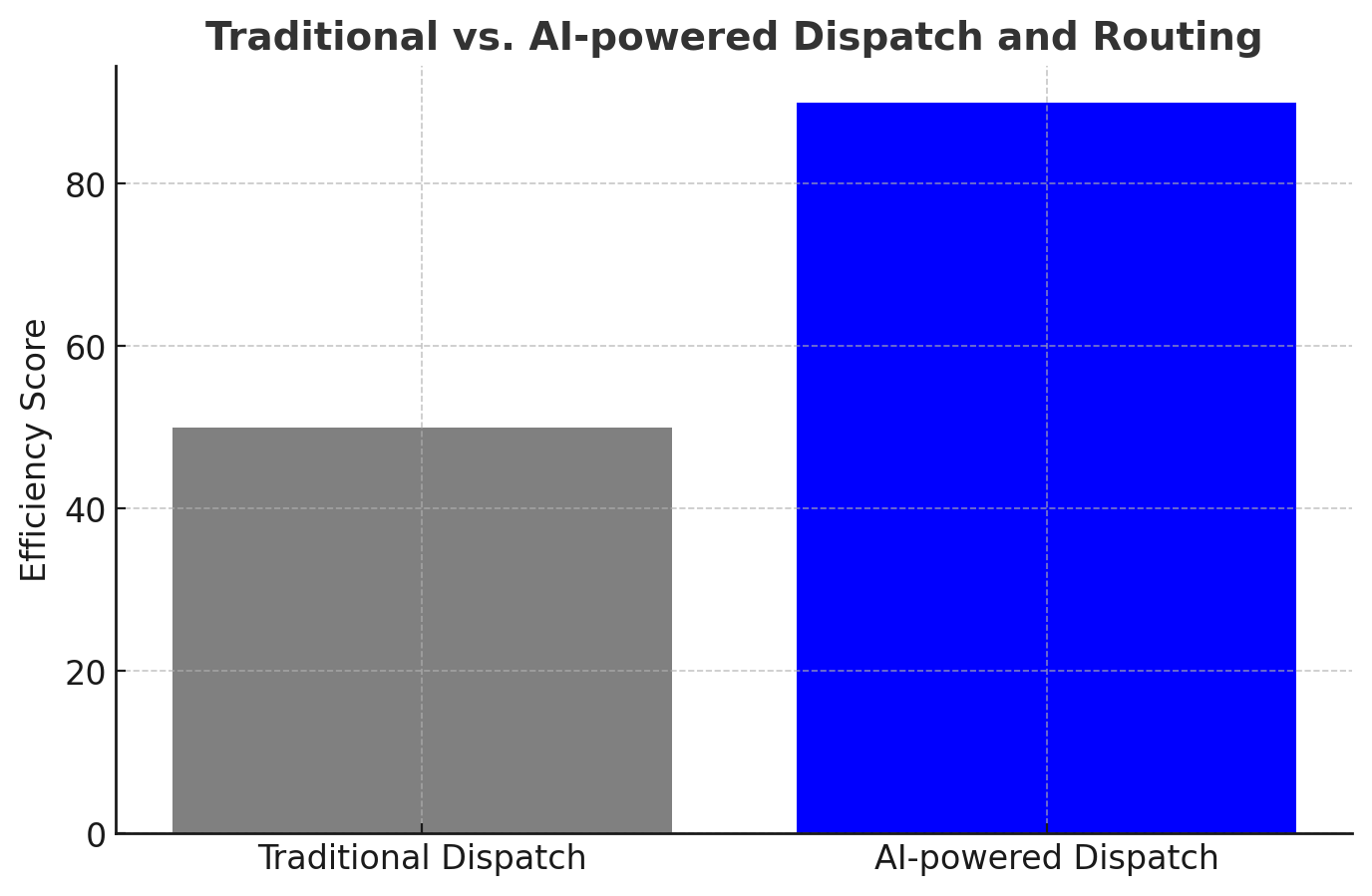
Before: Manual dispatch and routing systems
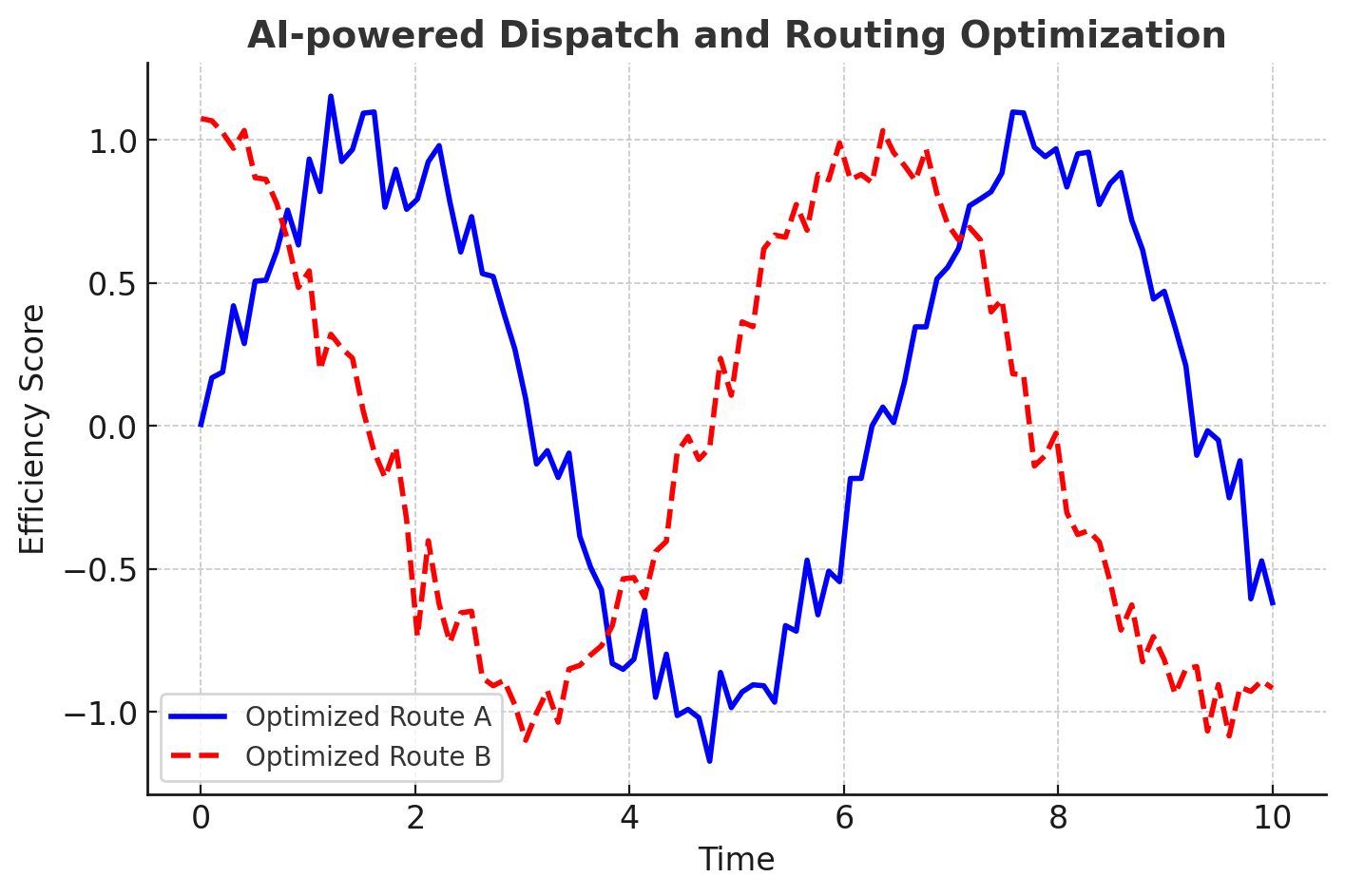
After: AI-powered dispatch and routing optimization
2. The Challenge
RxHealthline faced multiple challenges in delivering timely emergency medical services:
Extended Response Times
Average emergency response times of 25-30 minutes in urban areas, well beyond the critical golden hour window for many emergencies.
Inefficient Resource Allocation
Manual dispatch systems led to suboptimal ambulance deployment, with some areas overserved while others experienced critical shortages.
Traffic Congestion
Dense urban traffic patterns significantly delayed ambulance arrival times, with no real-time routing optimization.
Limited Predictive Capabilities
Inability to predict emergency hotspots and optimize ambulance positioning proactively.
Suboptimal Patient Outcomes
Delayed response times resulted in poorer patient outcomes, particularly for time-sensitive emergencies like cardiac arrests and strokes.
These challenges resulted in a significant number of preventable deaths and complications, with RxHealthline struggling to meet their mission of providing timely, life-saving emergency care.
3. Our Solution
Vinkura AI developed a comprehensive AI-powered emergency response system specifically designed to address RxHealthline's challenges and optimize the critical golden hour window.
Key Components
- 1
AI-Powered Dispatch System
Intelligent emergency call analysis and ambulance assignment based on severity, location, and available resources.
- 2
Dynamic Routing Optimization
Real-time route calculation considering traffic conditions, road closures, and shortest paths to appropriate medical facilities.
- 3
Predictive Positioning
AI algorithms that predict emergency hotspots based on historical data, time of day, events, and weather conditions.
- 4
Hospital Capacity Management
Real-time tracking of hospital capacity and specialty availability to direct patients to the most appropriate facilities.
Key Features
- ✓
Golden Hour Optimization
Prioritization system that maximizes the number of patients receiving care within the critical first hour.
- ✓
En-Route Medical Guidance
AI-assisted guidance for paramedics based on patient symptoms and vital signs during transport.
- ✓
Resource Optimization
Intelligent allocation of ambulances, equipment, and medical personnel based on emergency type and severity.
- ✓
Preventive Care Integration
Connection with wellness monitoring systems to identify high-risk patients and provide preventive interventions.
4. Implementation
The implementation of Vinkura AI's emergency response system for RxHealthline was carried out in phases over a six-month period, ensuring minimal disruption to existing emergency services while gradually introducing the new technology.
Implementation Timeline
Phase 1: Assessment & Planning (1 Month)
• Analyzed existing emergency response data and identified key bottlenecks
• Mapped emergency patterns across different regions and time periods
• Developed implementation roadmap with RxHealthline leadership
• Established baseline metrics for measuring improvement
Phase 2: Pilot Deployment (2 Months)
• Deployed AI dispatch system in two high-volume urban districts
• Equipped 25 ambulances with real-time routing and medical guidance systems
• Trained dispatch personnel and paramedics on the new technology
• Gathered feedback and refined the system based on real-world usage
Phase 3: Full Deployment (2 Months)
• Expanded the system to all 150 ambulances in RxHealthline's fleet
• Integrated with hospital systems for real-time capacity management
• Implemented predictive positioning across all service areas
• Established 24/7 technical support and monitoring team
Phase 4: Optimization & Expansion (1 Month)
• Fine-tuned AI algorithms based on accumulated operational data
• Added preventive care integration with wellness monitoring systems
• Expanded analytics capabilities for continuous improvement
• Developed training program for new staff and ongoing education
Challenges & Solutions
Data Integration
Challenge: Integrating with diverse hospital systems and legacy emergency response infrastructure.
Solution: Developed flexible API connectors and middleware solutions to enable seamless data exchange while maintaining existing workflows.
Connectivity Issues
Challenge: Ensuring system reliability in areas with limited or intermittent connectivity.
Solution: Implemented edge computing capabilities in ambulances with offline functionality and automatic synchronization when connectivity is restored.
User Adoption
Challenge: Initial resistance from some paramedics and dispatch personnel accustomed to traditional methods.
Solution: Implemented a comprehensive training program with hands-on workshops and created a peer champion network to support adoption.
5. Results & Impact
After one year of full implementation, Vinkura AI's emergency response system delivered transformative results for RxHealthline and the communities they serve:
Response Time Improvements
Patient Outcomes
Additional Benefits
Operational Efficiency
- •41% improvement in ambulance utilization and resource allocation
- •32% reduction in operational costs through optimized routing and fuel usage
- •27% increase in service capacity without additional ambulances
Preventive Impact
- •18% reduction in emergency calls through preventive interventions
- •22% improvement in chronic condition management through early intervention
- •1,000+ high-risk patients enrolled in preventive monitoring programs
Transform Your Emergency Healthcare Services
Vinkura AI's emergency response system can be customized for healthcare providers of all sizes, delivering significant improvements in response times, patient outcomes, and operational efficiency.
Related Case Studies
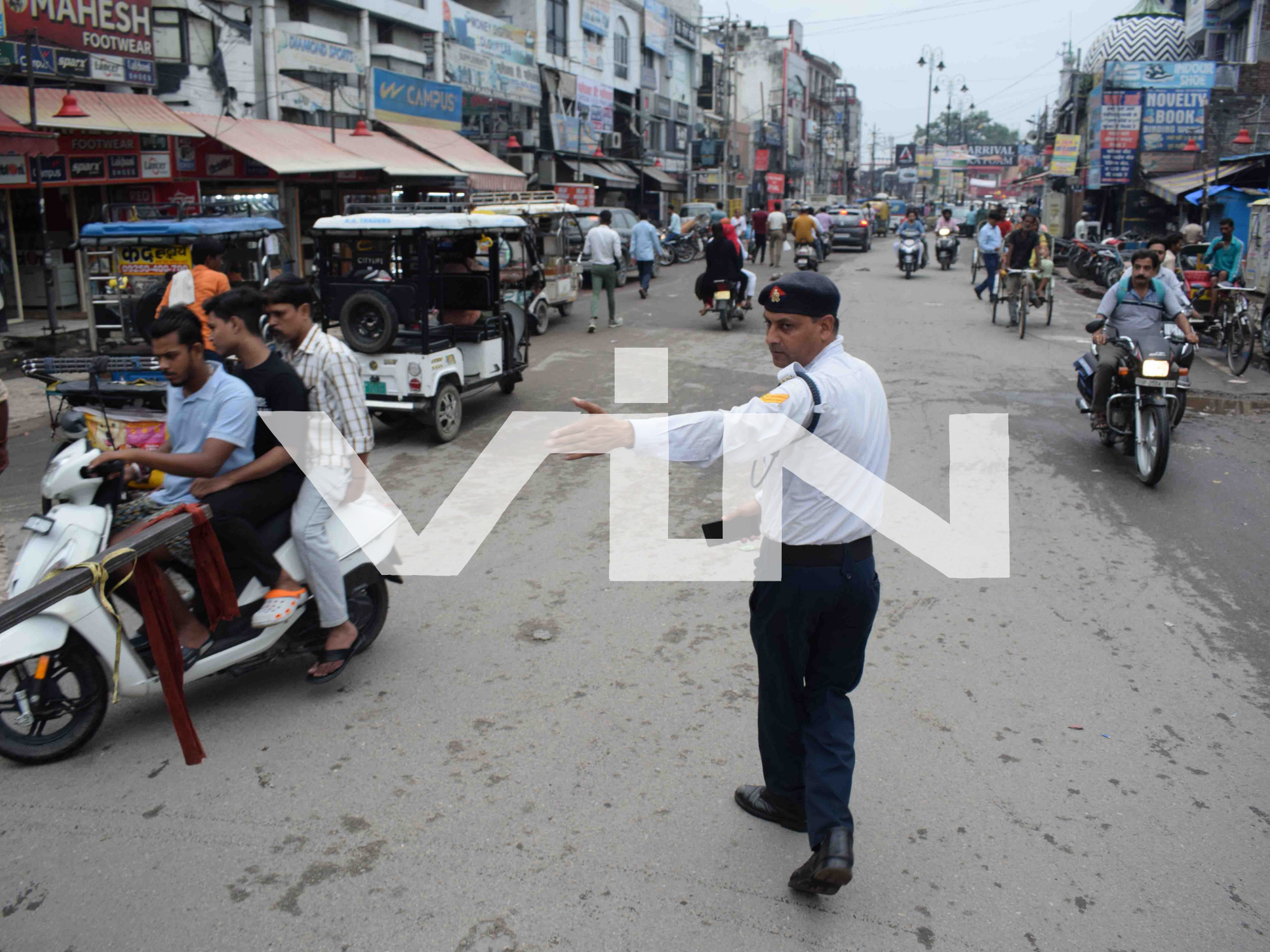
Decentralized AI for Traffic Management
How our AI solution reduced congestion by 32% and accidents by 27% in Bareilly.

AI-Powered Learning in Rural Schools
Transforming government schools in Bareilly with AI-driven personalized learning.
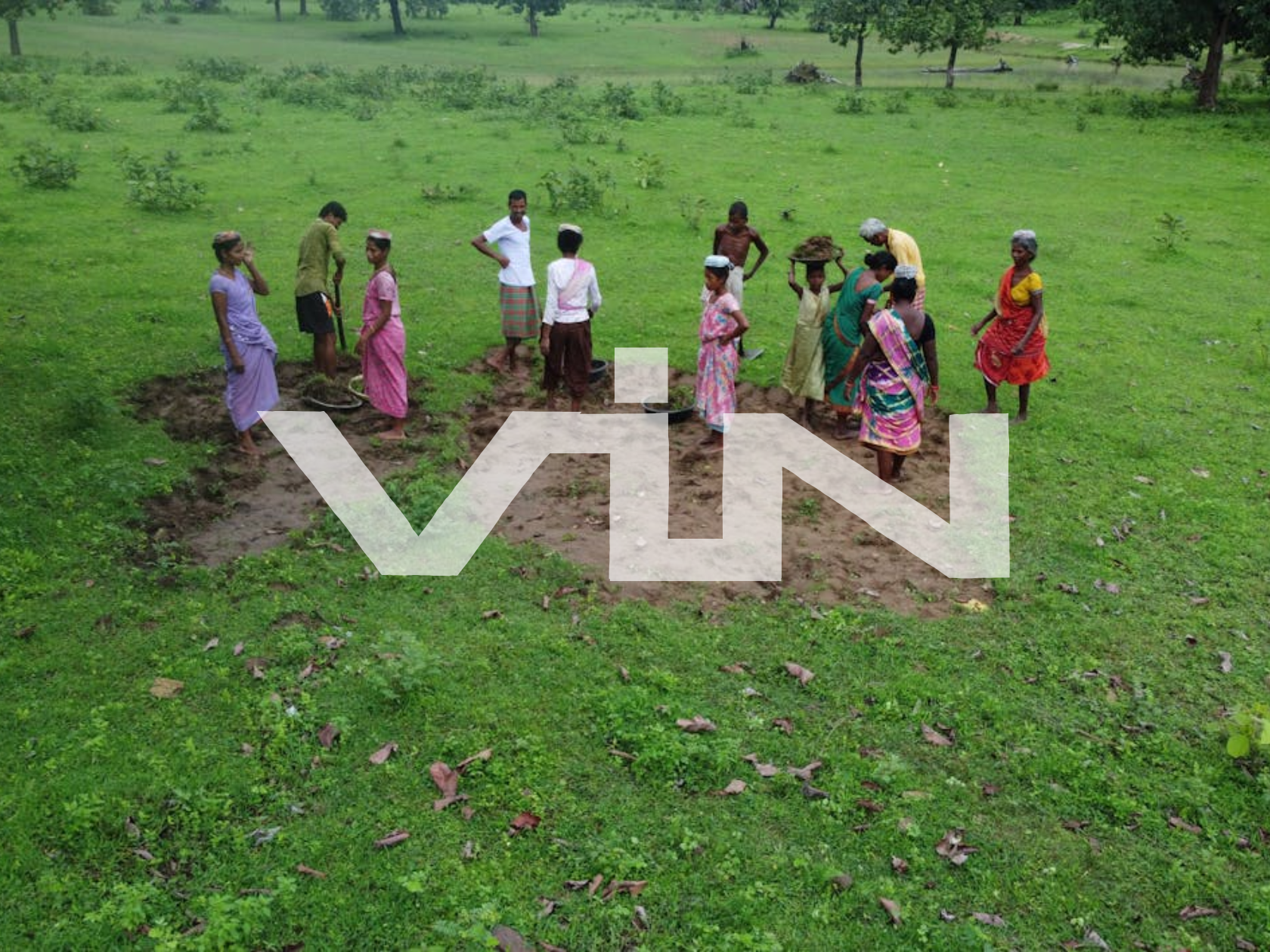
Smart Farming with Edge AI
How our AI solutions helped farmers increase crop yields and reduce resource usage.